Recent Advances in the Use of Exoglycosidases to Improve Structural Profiling of N-glycans from Biologic Drugs
Analytical exoglycosidases are transitioning from being largely academic tools to being suitable for glycan analytics in biopharmaceutical manufacturing.By Christopher H. Taron, Ian Walsh, Xiaofeng Shi, Pauline M. Rudd
Luk Cox/stock.adobe.com
Many classes of biologic drugs are recombinant secretory proteins (e.g., antibodies, fusion proteins, growth factors, cytokines) that carry post-translational carbohydrate (“glycan”) modifications. Glycans are typically found on certain asparagine residues (N-glycans) or serine/threonine residues (O-glycans). On a therapeutic protein, the composition of these glycans can significantly impact the drug’s stability, pharmacokinetics, and biological activity. Thus, glycan structure is a critical quality attribute (CQA) that must be monitored during drug manufacturing.
Glycan composition profiling is commonly used to track the structural diversity of oligosaccharides present on therapeutic proteins. A common approach to structural profiling involves first releasing glycans from a protein via chemical or enzymatic treatment (typically Peptide:N-glycosidase F [PNGase F] for N-glycans). Released glycans are then fluorescently labeled and subjected to solid-phase extraction to remove unreacted label (1). Fluorescently labeled N-glycans are commonly analyzed by separation using high- or ultra-high-performance liquid chromatography (HPLC or UHPLC, respectively) coupled to fluorescence detection (FLR), often with inline mass spectrometry (MS). Typically, dextran ladder mobility (GU values) and mass data (m/z) are collected for each chromatographed glycan peak, and their structures can be inferred by comparison to similar data from known N-glycans in reference databases such as GlycoStore (2).
Importantly, in some cases, highly similar glycan species cannot be discriminated solely by their mobility and mass (see Figure 1A). The problem arises from glycan species that co-migrate during UHPLC-FLR separation and have exactly the same mass (isomers) in the MS instrument. Co-migrating isomers are especially evident in complex samples such as serum (3) or plasma (4), in which a single UHPLC-FLR peak may contain over five glycan species with the same mass. In all samples, co-migrating isomers are best resolved by digestion with specific exoglycosidases.
Exoglycosidases are enzymes (hydrolases) that sequentially remove terminal monosaccharides from the non-reducing end of glycans (5). They typically have specificity for a particular monosaccharide, its anomeric configuration (α or β), and its position of attachment to an adjacent sugar within a larger oligosaccharide. They are powerful tools that can be used to modify the composition of glycans both in vitro or in situ(e.g., on the surface of a cell) (6). However, their most common use is in glycan structure determination (7–11). Several enzymes with precise specificities have emerged over many years as the glycobiology field’s preferred analytical exoglycosidases (see Figure 1B). These enzymes can be used individually or in combinatorial arrays, or panels, to elucidate a glycan’s structure (see Figure 1B, 1C). When used in conjunction with glycan profiling workflows, these enzymes play a crucial role in verification of the glycan structures present on a biologic drug or other glycoprotein.
Figure 1. Features of common analytical exoglycosidases. A) Shown are two N-glycan structures that are indistinguishable by mass and ultra-high-performance liquid chromatography (UHPLC) mobility data. In the example shown, treatment of each glycan with a-galactosidase (CBG) would remove one galactose from the structure on the right (changing its mass and UHPLC mobility), while the structure on the left would remain unaltered. B, C) Schematics illustrating how various analytical exoglycosidases can be used in a sequential manner (an array or panel) to remove each monosaccharide that comprises an N-glycan. C) Digested N-glycans are separated by UHPLC coupled to fluorescence detection (FLR) using hydrophobic interaction chromatography (HILIC), and monosaccharide removal events are visualized by peak shifts. Interpreting these shifts permits the entire structure to be experimentally verified. UND is undigested control. M3 is conserved trimannosyl core N-glycan.
While exoglycosidases ensure the highest accuracy in glycan structure determination, there have been several historical problems surrounding their routine use. There are critical issues involving poor manufacturing quality, supply inconsistencies, incompatibility with next-generation fluorophores (e.g., RapiFluor-MS), and lack of informatics resources that automate interpretation of exoglycosidase digestion data. This article summarizes several recent advances in glycoanalytics that have addressed many of the issues facing exoglycosidase use. These recent innovations are better positioning exoglycosidases for routine use in analytical workflows that affect biologic drug analysis.
New problems, new solutions
An ongoing pharmaceutical industry trend has been the adoption of faster and more sensitive glycoanalytical workflows. Over the past few years, several commercial suppliers (e.g., Waters, Prozyme, Ludger) have started offering kits that improve the speed and sensitivity of N-glycan sample preparation for glycan profiling. These kits also simplify and standardize glycan profiling workflows. Sample preparation technologies have seen multiple recent innovations (1). For example, the time needed to achieve enzymatic deglycosylation of monoclonal antibodies has been reduced by nearly two orders of magnitude. Certain products (e.g., Rapid PNGase F, New England Biolabs; GlycoWorks kit,Waters) achieve complete deglycosylation of monoclonal antibodies in 10 minutes compared to previous protocols that often give incomplete deglycosylation even after overnight incubation. Additionally, a new generation of fluorescent dyes (e.g., RapiFluor-MS, InstantAB, and InstantPC) have improved labeling speed and/or sensitivity over traditional labels (e.g., 2-aminobenazamide [2-AB], 2-aminobenzoic acid [2-AA], procainamide). These newer dyes use reactive N-hydroxysuccinimidyl (NHS)-activated carbamate chemistry to rapidly create a urea linkage between the dye and a glycosylamine moiety that is present on the glycan’s reducing end immediately following PNGase F hydrolysis (see Figure 2) (1,12). This reaction is significantly faster than reductive amination chemistry that has been used for more than two decades with traditional dyes like 2-AA and 2-AB. These new “rapid” dyes are becoming increasingly popular in many biopharma glycoprofiling workflows due to their inclusion in commercial sample preparation kits (e.g., GlycoWorks, Waters and GlycoPrep, Prozyme).
Over the past two years, multiple studies have described a problematic phenomenon associated with NHSactivated carbamate dyes and exoglycosidase performance (13–15). The presence of any of several different urealinked dyes (RapiFluor-MS, AQC, InstantAB, InstantPC) on the innermost core N-acetylglucosamine (GlcNAc) of a released N-glycan inhibits enzymatic removal of core α 1,6 fucose by bovine kidney fucosidase (BKF), the enzyme historically used to confirm the presence of this important moiety (see Figure 2) (13–15). Molecular modeling with a core fucosylated RapiFluor-MS labeled N-glycan suggested that rigidity of the label likely caused steric clashes within the BKF active site (13). However, this problem has been recently addressed in a study describing the discovery and characterization of an α-fucosidase from Omnitrophica bacterium (abbreviated as “OTF” in Figures 1B, 1C, and 2) (15). OTF efficiently removes core 1,6 fucose from N-glycans labeled with any of the new urea-linked dyes, in addition to traditional dyes like 2-AB, 2-AA, and procainamide. Thus, OTF is superior to BKF for N-glycan corefucose determination in glycan profiling workflows using NHS-activated carbamate dyes.
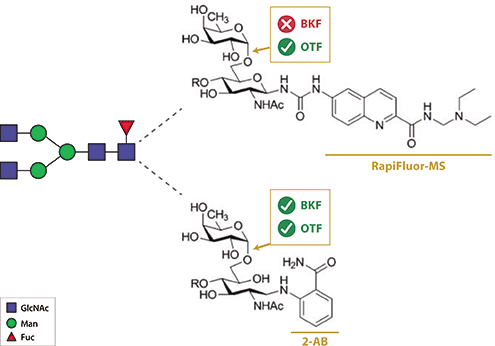
Figure 2. New N-glycan dyes inhibit the ability of bovine kidney fucosidase (BKF) to remove core a-1,6 fucose. The gray box on the N-glycan schematic denotes the region of the glycan enlarged into structural detail on the right. The top structure illustrates the urea-linked dye, RapiFluor-MS, attached to N-acetylglucosamine (GlcNAc), whose ring is closed. The bottom structure shows the traditional dye, 2-AB, attached to a GlcNAc with an open ring. The rigidity of RapiFluor-MS (and other similar dyes) renders BKF unable to remove fucose from the C6 position of GlcNAc. The new enzyme, OTF, is able to cleave fucose when either label is present on GlcNAc.
Advances in data interpretation
The use of panels or arrays of highly defined exoglycosidase specificities to “sequence” N-glycans was first described in the 1990s (8). In this method, a series of parallel exoglycosidase digestions is performed with enzymes that are specific for particular sugars that comprise N-glycans. Typically, the parallel reactions are individually analyzed using UHPLCFLR-MS and the glycan profiles are compared (Figure 1B,C). While this is a tremendously powerful analytical method, the need for manual analysis of the data generated has been a significant bottleneck. For example, a typical monoclonal antibody N-glycan sample may generate a dozen or more peaks in a UHPLC-FLR-MS profile. Each peak may or may not change upon treatment with an exoglysosidase. Thus, each N-glycan peak must be individually evaluated for its change in migration and mass for each enzyme used. When performed manually, such analysis is complicated and time-consuming.
A recent study computationally approached the problem of exoglycosidase array data interpretation (16). The result was a new web utility named GlycanAnalyzer, developed in a collaboration between New England Biolabs and the Bioprocessing Technology Institute (A*STAR, Singapore). The program automates annotation of UHPLC-FLR-MS profiles following digestion with one or more exoglycosidases. In this approach, users input tables of UHPLCFLR-MS mobility, intensity, and mass data (ideally) obtained during profiling analyses. Exoglycosidase digestions result in large numbers of peak movements in both the MS and UHPLC-FLR data, a major complicating factor for manual data interpretation. GlycanAnalyzer overcomes this by computing mass and mobility changes for each chromatographed peak upon enzyme treatment and pattern matches the results against values for known structures present in the public database GlycoStore (2). A score is generated to approximate the level of agreement between observed mass/mobility data and that of reference glycans, with a low score indicating good agreement. The program outputs data for each peak (see Figure 3) and reduces the time for data interpretation. Currently, the program only annotates N-glycan UHPLC-FLR-MS data, but future extended versions will permit structural assignment of other glycan moieties. In the coming years, it is anticipated that similar capabilities will become the norm in software packages associated with commercial glycoanalytical platforms.
Figure 3. Example of GlycanAnalyzer data output. A) Candidate N-glycan structures predicted for each peak are ranked by score (closer to zero is better). B) A user can accept or reject any candidate structure. C) Structures are given in Oxford text notation and D) drawn in context-free grammar notation. E) Comparisons between observed and theoretical masses and mobilities are given to aid annotation decisions. F) Links to reference databases are provided. G) Accepting a structure into the chromatogram increments according to the number of annotated peaks. Clicking on view chromatogram gives a summary of currently annotated peaks. H) Links to other graphics give evidence of peak movements and how sugar cleavage events contributed to the score.
Improved exoglycosidase manufacturing quality
Commercial manufacturing of analytical glycosidases has not significantly changed in nearly two decades. Many important analytical glycosidases, originally isolated for use in academic research in the 1980s, are still being produced as non-recombinant proteins purified from ground plant-seed meals (jack beans, almonds, coffee beans) or animal tissues (bovine testes, bovine kidney). These sources are well known for containing contaminating enzyme activities that are detrimental to modern ultrasensitive glycan analysis workflows. In recent testing of current commercial exoglycosidase preparations (17), it was shown that these enzymes are of poor physical purity (see Figure 4A) and many were contaminated with unwanted glycosidase specificities (see Figure 4B, C) that severely compromise their ability to be used for structure interpretation in glycan profiling. As such, there has been a critical need for significant improvement in glycosidase manufacturing processes.
Recently, New England Biolabs, a provider of recombinant and native enzymes for molecular biology applications, completed an effort to redesign manufacturing of the most widely used analytical glycosidases, with a goal of enabling their routine use in the analysis of biologic drugs and clinical diagnostic workflows (17). To accomplish this aim, several issues were addressed. First, recombinant production of all enzymes was achieved in Escherichia coli or yeast hosts. This required discovery of some genes encoding plant enzymes that had never been produced recombinantly (e.g., jack bean mannosidase, almond meal fucosidase). Second, scalable fermentation and downstream processing procedures were developed for each enzyme. These processes avoided the direct use of animal-derived components. Additionally, multiple production lots of each enzyme were produced to demonstrate process consistency and enable batch-to-batch comparisons. Third, new quality control assays were devised to ensure the absence of contaminating glycosidases and proteases. Finally, enzymes were manufactured under international quality management systems to ensure a high level of traceability and quality control.
Figure 4. A) Physical quality of commercial analytical exoglycosidases. Comparison of commercial preparations of common analytical glycosidases visualized by sodium dodecyl sulfate-polyacrylamide gel electrophoresis separation followed by staining. The empty lane for supplier 2 NAN1 represents separation of 20 L of commercial stock without protein observation. B) Contaminating b-galactosidase activity in commercial preparations of coffee bean a-galactosidase (CBG). C) Contaminating a-neuraminidase activity in commercial preparations of S. pneumoniae b-galactosidase (SPG). AMC is 7-amino-4-methylcoumarin (fluorophore). pNP is p-nitrophenyl (chromophore). Reactions were carried out according to each manufacturer recommendation and were incubated for 16 hours. Percent hydrolysis was calculated by comparison to a control reaction using an enzyme that fully hydrolyzed each substrate.
Summary
Analytical exoglycosidases are transitioning from being largely academic tools to being suitable for glycan analytics in industries where consistency and accuracy are of paramount importance. New specificities and computational methods to automate exoglycosidase data interpretation are working in conjunction with modern, highly sensitive analytical workflows to improve glycan profiling of biologic drugs. Glycans annotated using these innovative methods have higher structural details, down to the position and isomeric linkage of each monosaccharide. Additionally, substantial improvements to exoglycosidase manufacturing process and quality are enabling these enzymes to play important roles in other clinically important areas, such as cellular or protein glycoengineering, vaccine development, and molecular diagnostics.
Acknowledgements
The authors thank Barbara Taron for critical review of the article and Tasha José for assistance with graphics.
References
1. R. Duke and C. H. Taron, BioPharm Int. 28 (12), 59–64 (2015).
2. S. Zhao, et. al, Bioinformatics online, DOI: 10.1093/bioinformatics/bty319 Apr. 20, 2018.
3. R. Saldova, et. al, Mol. Oncol. 11 (10), 1361-1379 (2017).
4. M. Doherty, et. al, Sci. Rep. 8 (1) 8655 (2018).
5. A. Kobata, Proc. Jpn. Acad., Ser B. 89 (3), 97-117 (2013).
6. E. P. Guthrie and P.E. Magnelli, Bioprocess Int. 14 (2016).
7. A. Kobata, Anal. Biochem. 100, 1–14 (1979).
8. P. M. Rudd, et. al, Nature 388, 205-207 (1997).
9. S. Prime and T. Merry, Methods Mol. Biol. 76, 53–69 (1998).
10. L. Royle, et. al, Methods Mol. Biol. 347, 125–143 (2006).
11. K. Mariño, et. al, Nat. Chem. Biol. 6, 713-723 (2010).
12. M. A. Lauber, et. al, Anal. Chem. 87 (10), 5401–5409 (2015).
13. R. O’Flaherty, et. al, J. Proteome Res. 16, 4237–4243 (2017).
14. M. Hilliard, et. al, MAbs 9, 1349–1359 (2017).
15. S. Vainauskas, et. al, Sci. Rep. 8 (1), 9504 (2018).
16. T. Walsh, et. al, Bioinformatics online, DOI: 10.1093/bioinformatics/bty681, Aug. 7, 2018.
17. C. H. Taron, et. al, NEB White Papers, DOI: 10.13140/RG.2.2.27258.95684 Aug. 1, 2017.
About the authors
Christopher H. Taron*is scientific director, Protein Expression and Modification Division, taron@neb.com, Xiaofeng Shi is development group leader; both are at New England Biolabs. Pauline M. Rudd is a visiting investigator, and Ian Walsh is an associate staff scientist; both are at Bioprocessing Technology Institute, Agency for Science, Technology and Research.
*To whom correspondence should be addressed.